How Machine Learning is Transforming Semiconductor Manufacturing Processes
The semiconductor industry, a cornerstone of modern technology, is undergoing a transformation fueled by machine learning (ML) and artificial intelligence (AI). These technologies have become essential in optimizing manufacturing processes, driving efficiency, and ensuring quality. From chip design to defect detection, AI/ML for semiconductors is revolutionizing how we approach production and problem-solving. Central to these advancements is the SECS/GEM protocol, a communication standard that facilitates seamless integration of ML systems into semiconductor manufacturing equipment.
The Role of Machine Learning in Semiconductor Manufacturing
Machine learning in semiconductor manufacturing plays a pivotal role in tackling complex challenges. Traditional methods often struggle to cope with the intricate processes involved in creating semiconductor devices. ML models, however, excel at analyzing vast amounts of data, identifying patterns, and making predictions that improve decision-making.
One key application is machine learning in chip design, where algorithms assist in optimizing layouts and detecting potential issues before physical prototypes are created. This accelerates the design process and reduces costs. Additionally, AI for yield prediction leverages historical production data to forecast outcomes, helping manufacturers fine-tune processes and minimize waste.
SECS/GEM, the Semiconductor Equipment Communications Standard/Generic Equipment Model, supports this technological shift by enabling real-time data exchange between manufacturing equipment and ML systems. This ensures that the insights derived from ML models can be swiftly implemented, enhancing productivity and consistency.
Enhancing Defect Detection with AI
Defect detection is another area where AI in semiconductor manufacturing has proven invaluable. The production of semiconductor devices requires extreme precision, and even minor defects can compromise functionality. Traditional inspection methods are time-consuming and prone to human error. Machine learning models, on the other hand, can analyze high-resolution images and identify anomalies with unparalleled accuracy.
For example, deep learning algorithms trained on image datasets can detect microscopic defects during wafer inspection, significantly reducing downtime and material waste. SECS/GEM protocols facilitate the integration of these AI systems with existing inspection equipment, allowing manufacturers to automate defect detection and ensure consistent quality.
Furthermore, AI in lithography has emerged as a game-changer. Lithography, the process of transferring patterns onto silicon wafers, is a critical step in semiconductor manufacturing. ML algorithms optimize the parameters of lithography equipment, ensuring precise patterning and minimizing errors. This reduces rework and improves throughput, saving both time and resources.
Process Optimization and Quality Assurance
Process control and quality assurance are integral to semiconductor manufacturing, and machine learning enhances these areas significantly. ML models analyze data from various stages of production to identify deviations and suggest corrective actions. This is particularly crucial in advanced manufacturing environments where even slight variations can lead to defective products.
AI/ML for semiconductors also extends to process optimization. By leveraging real-time data and predictive analytics, manufacturers can adjust parameters dynamically to maintain optimal conditions. The SECS/GEM standard plays a critical role here, enabling seamless communication between ML systems and manufacturing tools, ensuring that adjustments are executed in real time.
Conclusion
Machine learning is undoubtedly transforming semiconductor manufacturing processes. From AI for yield prediction to machine learning in chip design, the applications are vast and impactful. The integration of AI systems with manufacturing equipment, supported by protocols like SECS/GEM, ensures that these technologies are effectively implemented, driving efficiency, reducing costs, and maintaining quality.
As the semiconductor industry continues to evolve, the role of AI/ML for semiconductors will only expand. By embracing these advancements, manufacturers can stay ahead in a highly competitive market, delivering innovative and reliable products that power the digital age.
Featured Product
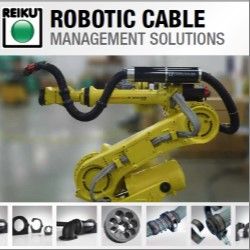