AI-Powered Semiconductor Process Control Systems: Revolutionizing Efficiency and Precision
The semiconductor industry has long been a cornerstone of technological advancement, powering everything from smartphones to advanced AI systems. However, the complexity of semiconductor manufacturing continues to grow, requiring increasingly sophisticated tools to meet the rising demand for efficiency, precision, and innovation. Enter AI-powered semiconductor process control systems, which are revolutionizing the way the industry operates by improving yields, reducing costs, and driving process optimization.
The semiconductor industry has long been a cornerstone of technological advancement, powering everything from smartphones to advanced AI systems. However, the complexity of semiconductor manufacturing continues to grow, requiring increasingly sophisticated tools to meet the rising demand for efficiency, precision, and innovation. Enter AI-powered semiconductor process control systems, which are revolutionizing the way the industry operates by improving yields, reducing costs, and driving process optimization.
In this blog, we'll explore how AI is transforming semiconductor process control and why it's crucial for the future of semiconductor manufacturing.
The Challenges of Semiconductor Manufacturing
Semiconductor manufacturing is an incredibly intricate process that involves numerous steps, each of which requires precise control to ensure the final product meets stringent quality standards. From wafer fabrication to etching, deposition, and packaging, every step must be carefully monitored and controlled.
A slight variation in any step can lead to significant defects, which results in yield loss, higher costs, and longer production times. Traditionally, human operators, as well as rule-based systems, have been employed to monitor these processes. However, as the complexity of chip designs has grown and technology scales down (think nanometers), traditional methods are no longer sufficient to maintain the required precision.
This is where AI-powered process control systems come into play. AI systems can analyze massive amounts of data from various sensors and tools in real-time to detect trends, predict outcomes, and optimize production processes.
The Role of AI in Semiconductor Process Control
AI-powered systems utilize a combination of machine learning (ML) algorithms and deep learning techniques to analyze real-time data and make decisions autonomously. Let's explore how AI is making a significant impact in several key areas of semiconductor manufacturing.
1. Real-Time Data Monitoring and Analysis
One of the biggest advantages of AI in semiconductor process control is its ability to process vast amounts of data from various sensors and tools across the manufacturing floor. Traditional monitoring systems are often limited in terms of the data they can analyze or their ability to make sense of complex relationships between different variables.
AI, on the other hand, is designed to handle large datasets and can monitor real-time sensor data continuously. By analyzing the data in real-time, AI systems can identify minor deviations or anomalies in the manufacturing process and alert operators before a defect occurs. This reduces the risk of yield loss and increases efficiency.
2. Predictive Maintenance
The reliability of semiconductor equipment is paramount to maintaining production efficiency. Traditional maintenance practices are often based on fixed schedules or reactive approaches, which may not always be the most efficient method for preventing equipment failures.
AI-enabled predictive maintenance leverages machine learning algorithms to analyze data from various sensors embedded in equipment to predict when maintenance is required. By analyzing patterns in data such as temperature, pressure, or vibration, AI can detect early signs of wear and tear that might not be visible to the human eye. This enables semiconductor manufacturers to schedule maintenance proactively, preventing unplanned downtime and minimizing the risk of equipment failure.
3. Process Optimization
AI-powered process control systems play a pivotal role in optimizing the semiconductor manufacturing process. Through continuous learning from historical and real-time data, AI systems can fine-tune various process parameters, such as temperature, pressure, or chemical composition, to improve yields and reduce defects.
AI can also identify patterns or combinations of variables that result in suboptimal performance and suggest adjustments that operators may not have otherwise noticed. These insights can lead to improved efficiency, reduced cycle times, and better overall process control. In a fast-paced environment like semiconductor manufacturing, AI's ability to continuously optimize processes is invaluable.
4. Quality Control and Defect Detection
In semiconductor manufacturing, even the smallest defects can lead to significant performance issues. Quality control is essential, but inspecting every wafer or chip manually is not only time-consuming but also prone to human error.
AI-powered computer vision systems, trained using machine learning algorithms, are being used to automate defect detection. By analyzing high-resolution images of wafers and chips, AI systems can identify defects like cracks, contamination, or pattern irregularities with greater accuracy than human inspectors. This leads to faster defect detection, fewer false positives, and, ultimately, higher-quality products.
5. Yield Prediction and Analysis
Yield, or the percentage of functional semiconductor chips produced per wafer, is a critical metric in semiconductor manufacturing. Improving yield is essential to maximizing profitability and reducing waste. Traditional methods of yield prediction often rely on historical data and fixed parameters, but these can be limited in terms of accuracy.
AI algorithms, however, can analyze real-time data from the manufacturing process to predict yield outcomes with greater precision. By identifying the factors that impact yield, such as process conditions, material properties, and machine performance, AI can offer valuable insights to improve production strategies and increase overall yield.
The Future of AI in Semiconductor Process Control
The future of AI in semiconductor process control looks promising, as machine learning and deep learning technologies continue to evolve. As AI systems become more advanced, they will be able to handle even more complex manufacturing processes, further enhancing efficiency, reducing waste, and improving quality.
AI is expected to play an even larger role in the automation of semiconductor manufacturing, helping manufacturers meet the growing demand for faster, more powerful chips while maintaining high standards of quality. As AI models continue to learn from real-time data, their predictive capabilities will become increasingly accurate, driving even greater efficiencies across the industry.
AI-powered semiconductor process control systems are already making a significant impact on the semiconductor manufacturing industry. By improving real-time data monitoring, enabling predictive maintenance, optimizing processes, enhancing quality control, and providing better yield predictions, AI is helping semiconductor manufacturers reduce costs, improve efficiency, and deliver higher-quality products.
As the semiconductor industry continues to advance, AI will undoubtedly be a critical component in ensuring that manufacturers can meet the demands of an increasingly digital world. For companies looking to stay competitive in this fast-paced environment, embracing AI-powered solutions will be key to driving the next generation of semiconductor innovations.
Featured Product
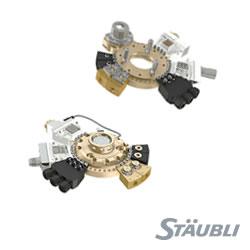